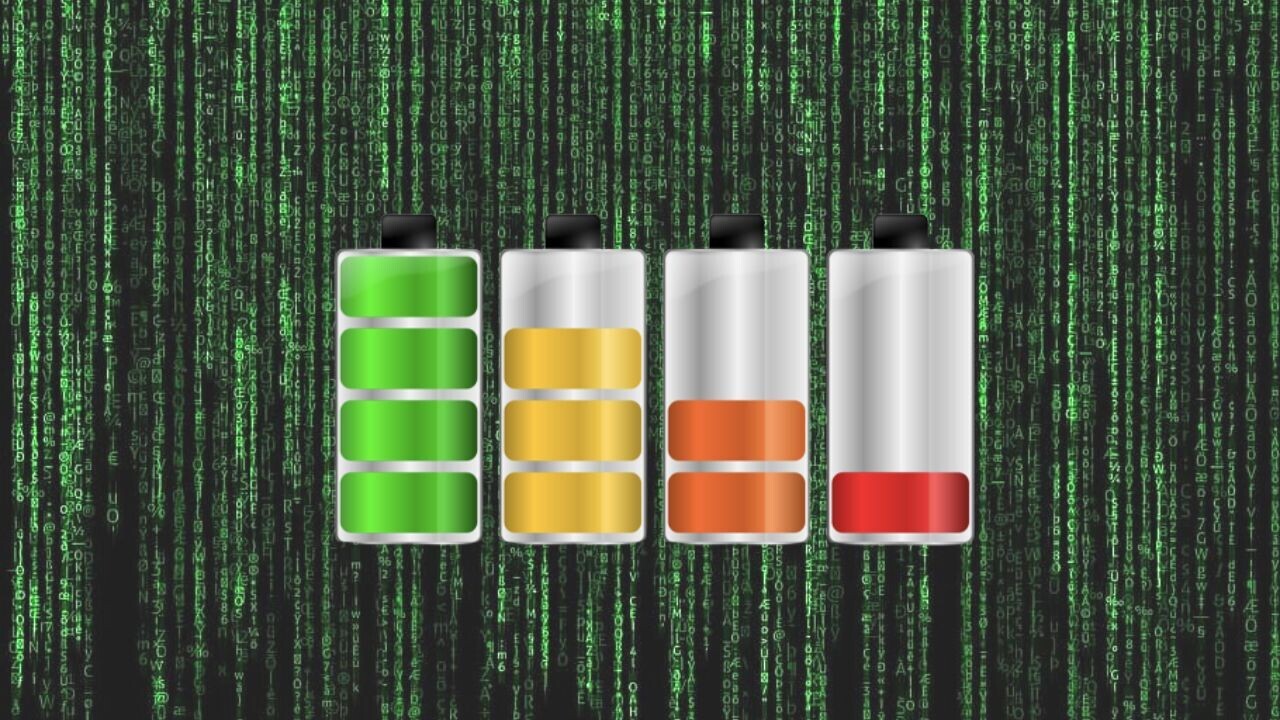
Lithium-ion batteries have become a key component in the rise of electric mobility, but forecasting their health and lifespans is limiting the technology.
While they’ve proven successful, the capacity of lithium-ion batteries degrades over time, and not just because of the ageing process that occurs during charging and discharging — known as “cycling ageing.”
Batteries also degrade when not in use
Lithium-ion battery cells also suffer degradation from so-called “calendar ageing,” which occurs during storage, or simply when the battery is not in use. It’s determined by three main factors: the rest state of charge (SOC), the rest temperature, and the duration of the rest time of a battery.
Given that an electric vehicle will spend most of its life parked, predicting the cells’ capacity degradation from calendar ageing is crucial; it can prolong battery life and pave the way for mechanisms that could even circumvent the phenomenon.
For this reason, researchers have been employing advanced machine learning algorithms to accurately predict calendar ageing.
Bridging research with the EV market
In a recent study funded by the EU’s Horizon 2020 program, a team of scientists took the research a step further by comparing the accuracy of two algorithms on the wide spectrum of commercial lithium-ion battery chemistries.
Specifically, they drew calendar ageing data from six types of battery cell chemistries: Lithium Cobalt Oxide (LCO), Lithium Iron Phosphate (LIP), Lithium Manganese Oxide (LMO), Lithium Titanium Oxide (LTO), Nickel Cobalt Aluminum Oxide (NCA), and Nickel Manganese Cobalt Oxide (NMC).
These battery cells were calendar aged in temperature chambers at 50, 60, and 70 degrees Celsius, using high, medium, and low voltages.
To predict ageing, the team investigated the efficiency of two machine learning algorithms: Extreme Gradient Boosting (XGBoost) and an artificial neural network (ANN).
How do the algorithms work?
Both algorithms were chosen for their ability to yield reliable results, but they differ significantly in their operation.
XGBoost is a decision tree-based, state-of-the-art supervised machine learning algorithm that’s widely used in regression or classification problems.
The ANN is an artificial adaptive system that uses its base elements, called neurons and connections, to transform its global inputs into a predicted output.
To evaluate their performance, the researchers used the mean absolute percentage error (MAPE) metric, which measures the average magnitude of errors between predicted and measured values. Simply put, the smaller the MAPE value, the higher the prediction accuracy.
What did the results show?
The algorithms’ testing showed that XGBoost can be used to effectively predict the calendar ageing of most chemistries with significantly minimal mean absolute error. Meanwhile, ANN produces satisfactory results for only the LFP, LTO, and NCA cell chemistries.
You can check out their accuracy in the graph below:
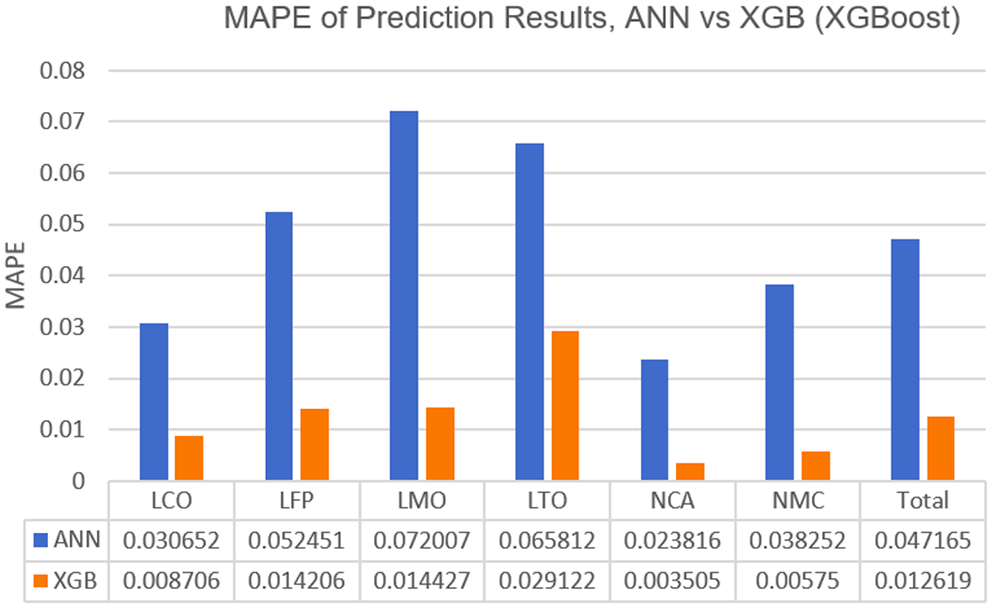
XGB’s overall superior performance, and in particular regarding battery chemistries that dominate the automotive industry (NCA, NMC, LFP), shows it could be incorporated into EV battery application softwares to successfully predict calendar ageing effects and provide better operation life to electric vehicle batteries.
It now remains to be seen which steps need to be taken to turn the research findings into commercial applications.
You can find the full research here.
Get the TNW newsletter
Get the most important tech news in your inbox each week.