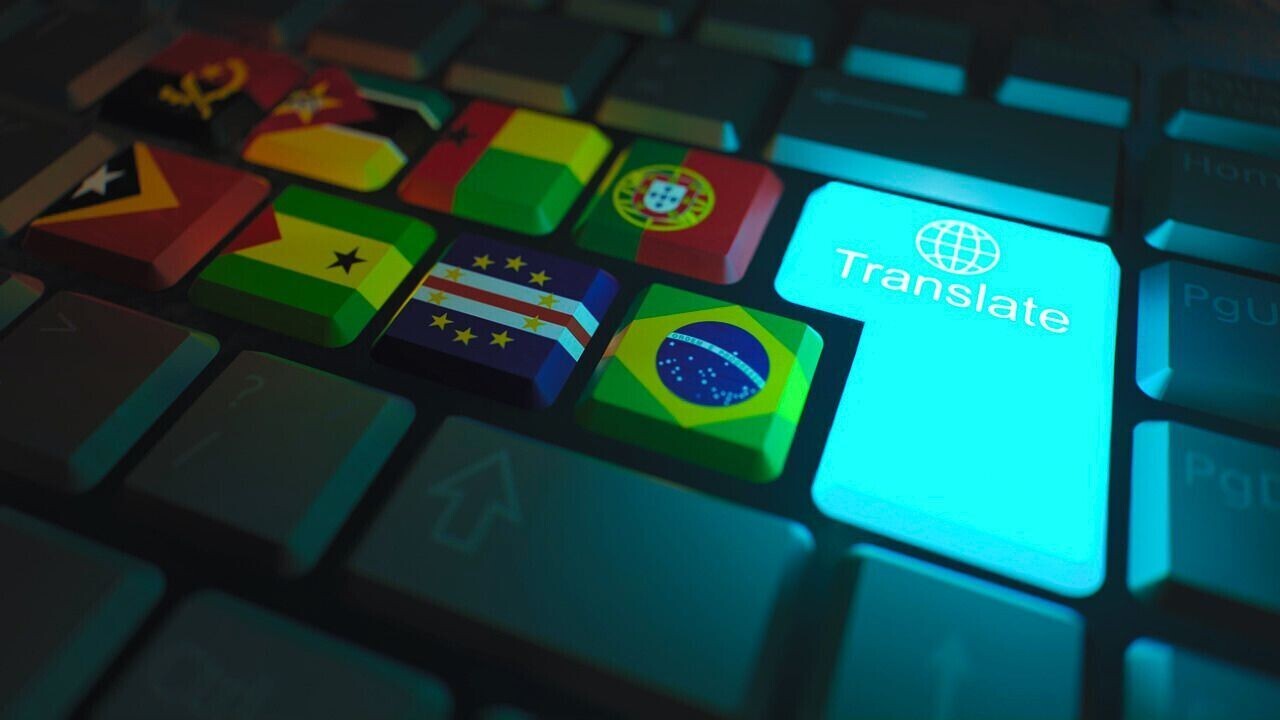
AI translation has transformed the way we communicate, breaking down language barriers in an unprecedented way. The sector’s global market size is projected to reach $12.3bn (€11.3bn) by 2026 — and big and small players alike are aiming to cash in.
Among them, Cologne-based DeepL has been raising industry standards even compared to tech giants like Google Translate and Microsoft Translator.
“We’ve always been rivalling big companies.
The startup was born from online dictionary Linguee and has grown fast since its founding in 2017 by Jarek Kutylowski, a computer scientist who’s also serving as the company’s CEO.
Born in Poland, Kutylowski moved to Germany at the age of 12 where he attended school without speaking a word of German. This made him realise the importance of language and the difficulty in having to communicate in more than one.
When he began working on DeepL in 2017, he saw that neural networks might offer a breakthrough that would enable technology to solve this problem. “We kind of knew that machine translation was going to go in this direction. And we knew this was going to be immensely helpful. Seeing this opportunity, we thought ‘Hey, let’s build something great,’” Kutylowski tells TNW.
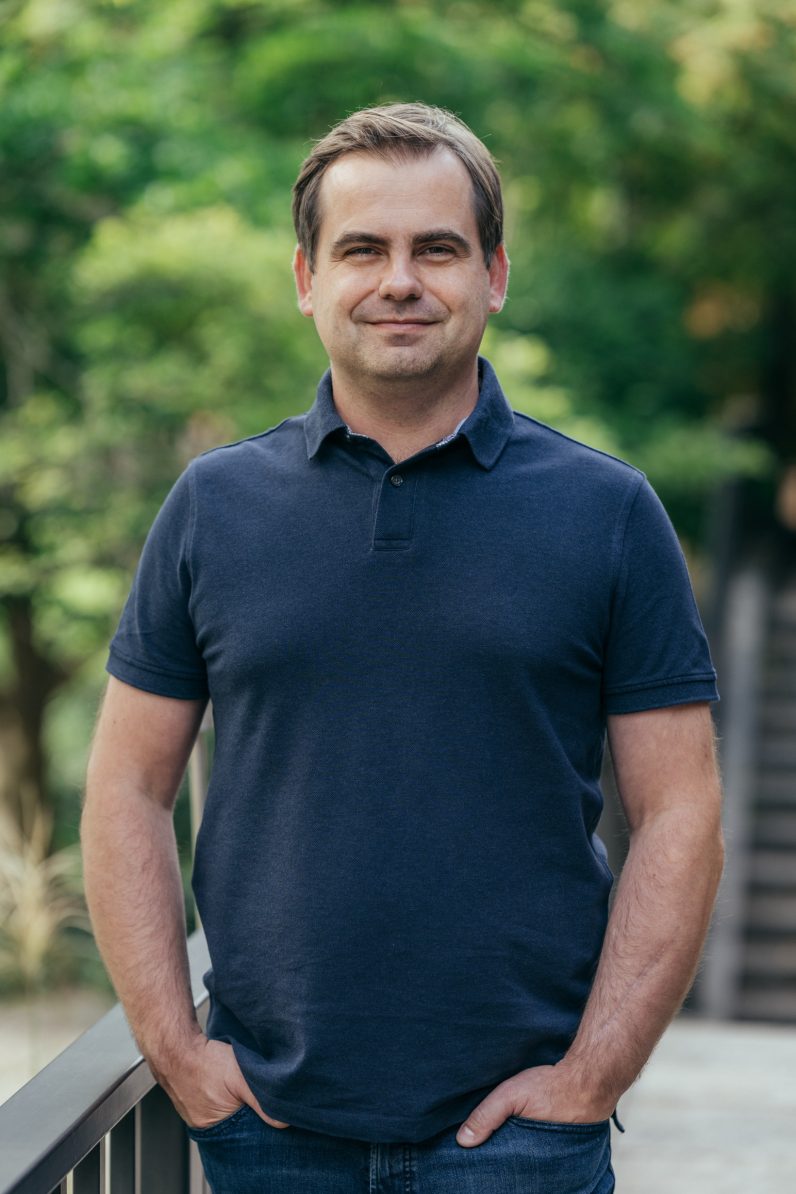
Neural Machine Translation (NMT) — as in, the one using neural networks — is the most successful machine translation method we have to date. Compared to its predecessors, it’s faster, more accurate, less resource-intensive, and easier to scale.
DeepL uses the technology to offer free and premium translation services, with special focus on B2B products. It says that, since its inception, over 1 billion users have made requests, and that it currently has more than 20,000 business customers, including the likes of Elsevier, Fujitsu, and Mastodon.
“Translation is really important for businesses,” Kutylowski explains. “Nowadays, companies start going global and expanding into other markets very quickly, so they get customers in different areas.”
He adds that the largest need for translation lies usually in those professions that are text-heavy, such as legal services. “This is where we see most often the strongest demand from our customer base,” Kutylowski says.
To date, DeepL covers 31 languages spanning across Europe and Asia. In 2023, it introduced its AI writing companion and secured unicorn status. Despite the tough funding environment, in January the company raised an undisclosed amount (estimated at €100mn), reaching a €1bn valuation.
The “world’s best” machine translation
DeepL makes the confident claim of offering the “world’s best” AI translation. In addition, it says its product is more nuanced and 3x more accurate compared to those of its competitors.
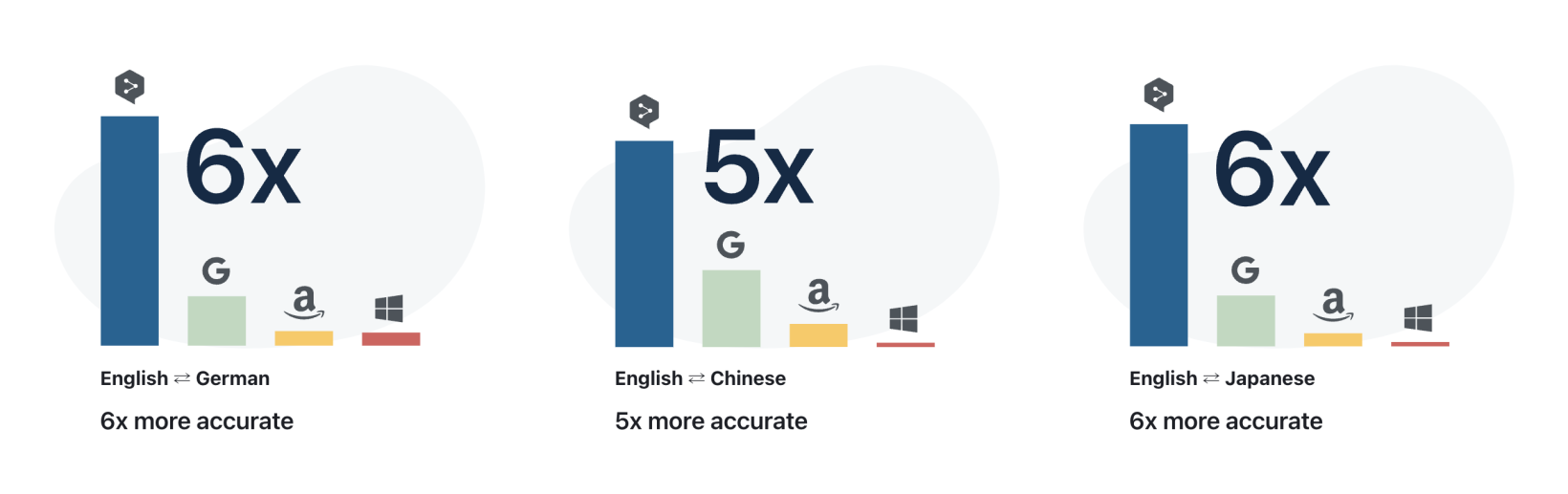
These assertions are based on “blind tests,” in which professional translators select the most accurate translation without knowing which company produced it.
When I ran a few test experiments of my own, DeepL did indeed come out on top. Firstly, I used a passage from The Stranger by Albert Camus and translated it from French into English, using bothDeepL’s translator and Google Translate.
Although the literary text category isn’t the purpose that these tools have been built for, I decided to begin with it anyway, as it is by default more difficult for an AI system.
That’s because the art of literary translation is complex and requires more than just linguistic proficiency. It involves a high level of creativity, a deep understanding of the author’s voice, style, and socio-historical background, as well as the transfer of meaning across different cultures.
Nevertheless, DeepL’s result was by far superior to Google’s. While it missed some uses of metaphorical language and made a few errors of intent and agreement, the end text did provide a closer meaning to the original.
I repeated the experiment using an article of my own to examine whether the translation tools conveyed the meaning I myself had intended. I translated from English into Greek (my native language).
Below is the DeepL translation:
And here is the Google Translate result:
Again, DeepL did a better job. Despite a couple of minor mistakes, the translation was more nuanced and natural in Greek, while also sticking to the original meaning. But since that’s probably all Greek to you, you don’t have to take my word for it, test it for yourself.
According to Kutylowski, conveying the right meaning to the target language without “butchering” it requires the right balance between accuracy and fluency. This heavily depends on the use case. For example, a technical document calls for higher accuracy, while a marketing text for higher fluency.
Despite this challenge, he believes that AI is capable of learning even the most complex languages. “If there was an alien language that we had to learn, nowadays, with the proper amount of material translated, we could probably work out the translation model for that too,” he adds.
What’s DeepL’s edge?
Kutylowski doesn’t seem concerned about the competition. “We’ve always been rivalling big companies,” he notes, adding that Google Translate remains DeepL’s biggest competitor.
He says that the startup’s edge comes down to a combination of three factors: hard work, a great team, and focus.
“Focus is always an important thing,” he says. “Translate isn’t the core business of Google — it’s one of the 100 side gigs. The same goes if you consider LLMs and the OpenAIs of this world as our competition; translation is only one thing of what they’re doing and their GPU is doing a tonne of different things. We’re focused on one particular area.”
From a technological perspective, DeepL’s success lies in the architecture of its neural networks, the input from human editors, and the training data.
The startup trains its models on tons of data, mostly from the internet, and employs special web crawlers to automatically find translations and assess their quality. It also uses methods such as reinforcement learning to provide positive feedback to the AI so it keeps producing the desired quality.
It’s also about finding the right balance between the capability of the model to translate and its capability to form sentences in the target language, Kutylowski adds. “So a lot of work goes into how much we are training the models on monolingual data and how much on translations. There are a lot of details which the mathematical team is taking care of.”
Machine translation: new challenges and opportunities
Kutylowski acknowledges that the recent boom in fascination with AI — to a great extent because of Large Language Models (LLMs) — has resulted in a more challenging and fast-paced landscape.
“Machine translation is a race now.
DeepL’s team now has to keep up to date with multiple developments: new models coming out, the open-source work that’s happening, academic research, and the work of other companies.
“Machine translation is kind of a race right now,” he says. So what’s a good strategy for competing in this race?
According to Kutylowski, one aspect is to be continuously innovating, and ensuring you’re taking the right steps to enable that. Investing appropriately is another. It also comes to securing the required capital and the right team.
But at the same time, the exponentially increasing interest and advancement in AI also brings new technological opportunities. “There are things that we thought about two or three years ago that technology wasn’t there yet to enable,” he says.
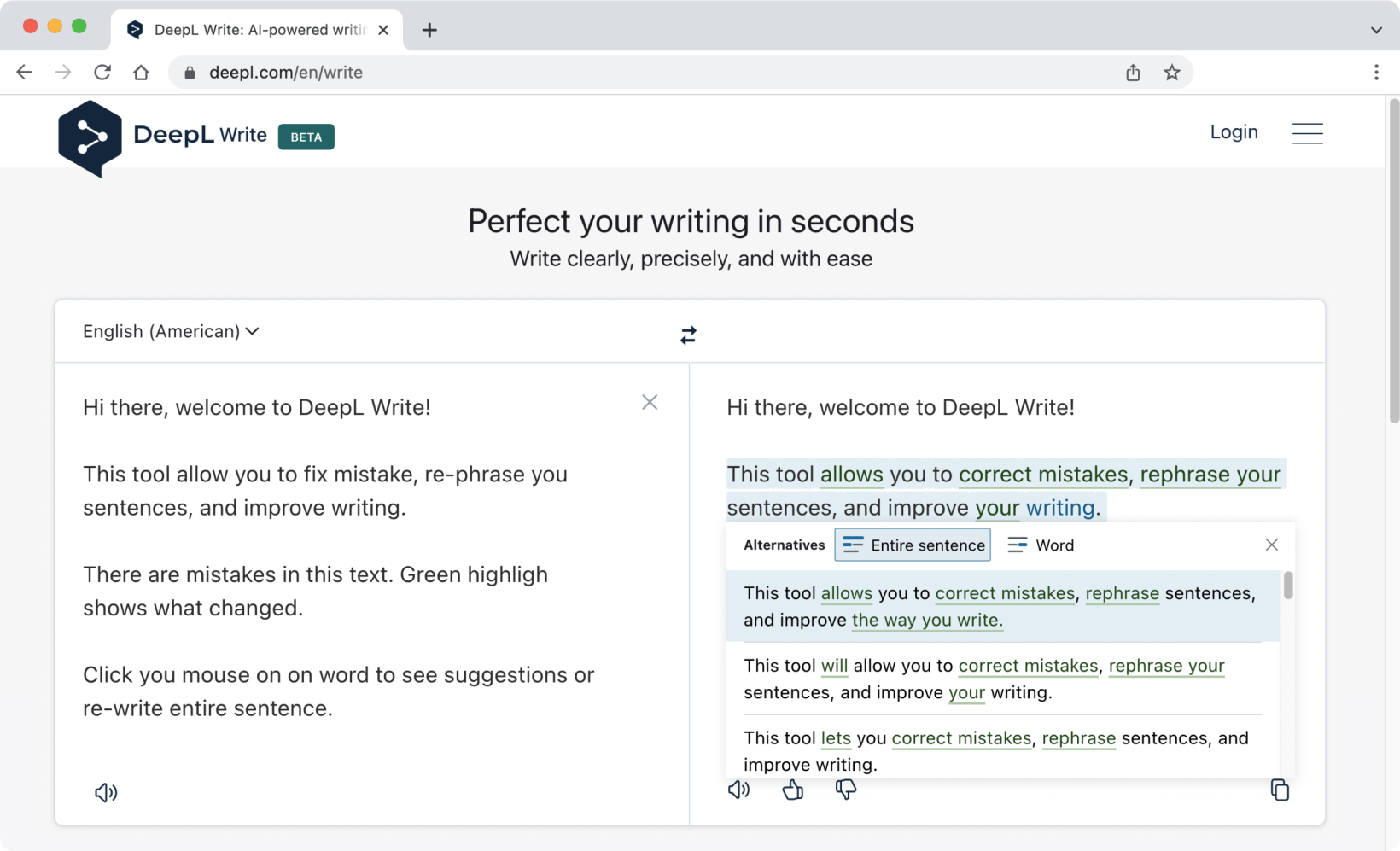
This includes personalised translations that fit a company’s style and a more interactive translation experience. DeepL has also begun research into invoice translation, while it’s training its own LLMs from scratch — in part, thanks to its new supercomputer cluster, DeepL Mercury.
These LLMs will open up opportunities to further improve translation quality and enable new, interactive workflows for users, with more capabilities and applications to be unveiled in 2024.
The future of language learning
Machine translation has had a tremendous impact on overcoming communication barriers. But this also bids the question: will we reach a point where we’ll no longer be learning foreign languages because AI can do it for us?
“With AI advancement in general, I think we as humans will have to ask ourselves the question, what do we need to learn? And what do we want to learn?,” Kutylowski says.
He believes that, when it comes to surviving in a foreign country, the necessity of language learning will gradually decrease as the technology gets better and better. But this doesn’t mean that the value in learning languages will decrease.
To illustrate, he uses maths as an example. While in real life we don’t apply the majority of the complex equations we were taught at school, the process of learning is still vital because it teaches us rational thinking.
The same goes for languages, Kutylowski says. When we learn a language we are also learning how to form thoughts and articulate ideas — and that’s crucial for our development.
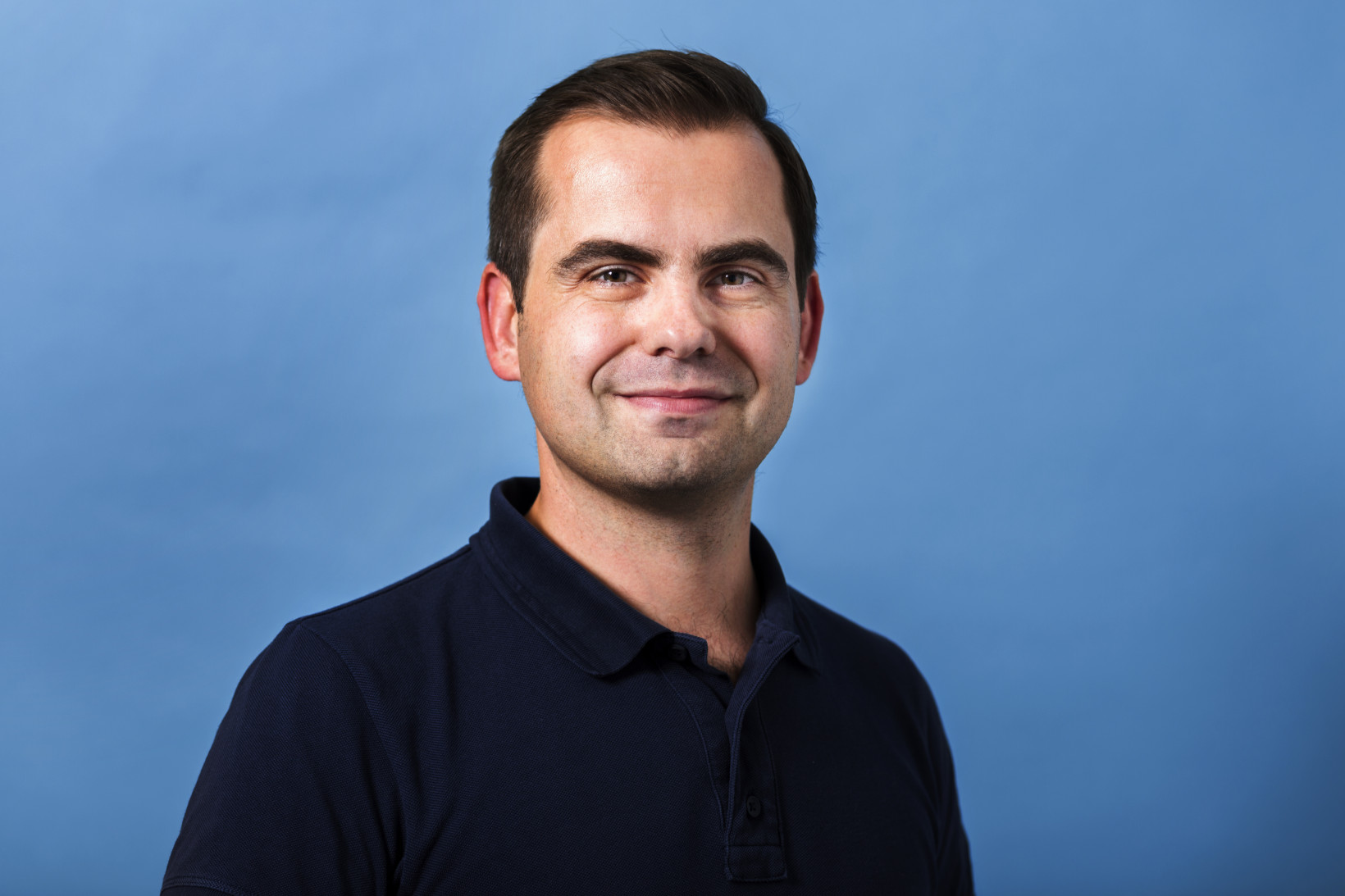
The benefits of foreign language learning and bilingualism are indeed far-reaching both for the individual and the society.
Research shows that learning a second language actually changes the brain. Specifically, it increases the density of grey matter (the number of neurons in the brain) as well as the integrity of white matter (the system of nerve fibres that connect the different regions of the brain). This not only strengthens overall brain function, but also enhances memory, attention, concentration, and other cognitive abilities.
In addition, numerous studies have linked language learning to better academic performance, higher employability, improved creativity, as well as communication skills and cross-cultural awareness.
“So for your own pleasure and for the development of your brain and personality, it’s still going to be important to learn languages,” Kutylowski notes. “And even with the best translator on your phone, if you’re marrying a partner who’s from a different country, you’re not going to be communicating through your phone. Or at least, I hope so.”
Get the TNW newsletter
Get the most important tech news in your inbox each week.